In this post we explore the basics of the Plumber package. Our aim is to ilustrate how to fit a \(L^2\)-regularized linear model and expose it to a web API so that we can request predictions.
Prepare Notebook
Let us load the necessary libraries.
library(caret)
library(httr)
library(magrittr)
library(plumber)
library(tidyverse)
Load Data
As a toy example we consider the mtcars
data set.
df <- mtcars %>% as_tibble()
df %>% head
## # A tibble: 6 x 11
## mpg cyl disp hp drat wt qsec vs am gear carb
## <dbl> <dbl> <dbl> <dbl> <dbl> <dbl> <dbl> <dbl> <dbl> <dbl> <dbl>
## 1 21 6 160 110 3.9 2.62 16.5 0 1 4 4
## 2 21 6 160 110 3.9 2.88 17.0 0 1 4 4
## 3 22.8 4 108 93 3.85 2.32 18.6 1 1 4 1
## 4 21.4 6 258 110 3.08 3.22 19.4 1 0 3 1
## 5 18.7 8 360 175 3.15 3.44 17.0 0 0 3 2
## 6 18.1 6 225 105 2.76 3.46 20.2 1 0 3 1
We want to fit a simple linear model to predict the variable mpg
.
Warning: We are not interested to find the “best model”. Better feature engineering and hyperparameter tunig is not developed here because it is not the main purpose.
Correlation Plot
For variable selection we consider a correlation plot.
df %>% cor %>% corrplot::corrplot()
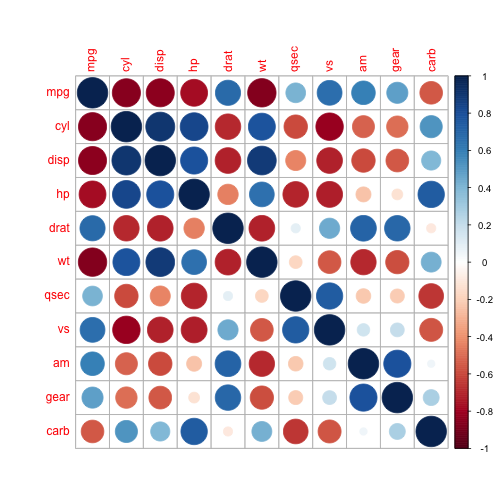
From the visualization we see that the variables wt
, qsec
and am
could be good predictors.
Define and Train Model
We are going to use the Caret package.
Split Data
set.seed(seed = 0)
# Define observation matrix.
X <- df %>% select(wt, qsec, am)
# Define target vector.
y <- df %>% pull(mpg)
# Define a partition of the data.
partition <- createDataPartition(y = y, p = 0.75, list = FALSE)
# Split the data into a training and test set.
df.train <- df[partition, ]
df.test <- df[- partition, ]
X.train <- df.train %>% select(wt, qsec, am)
y.train <- df.train %>% pull(mpg)
X.test <- df.test %>% select(wt, qsec, am)
y.test <- df.test %>% pull(mpg)
Data Pre-Processing
As we want to use a linear model, we neet to scale the variables.
# Define scaler object.
scaler.obj <- preProcess(x = X.train, method = c('center', 'scale'))
# Transform the data.
X.train.scaled <- predict(object = scaler.obj, newdata = X.train)
X.test.scaled <- predict(object = scaler.obj, newdata = X.test)
Train Model
We fit \(L^2\)-regularization linear model using a 3-fold cross-validation to tune the regularization hyperparameter.
model.obj <- train(x = X.train.scaled,
y = y.train,
method = 'ridge',
trControl = trainControl(method = 'cv', number = 3),
metric = 'RMSE')
Model Evaluation
Let us evaluate the model perforance.
On the training set:
model.obj$results %>% select(RMSE)
## RMSE
## 1 2.613844
## 2 2.613700
## 3 2.554912
On the test set:
y.pred <- predict(model.obj, newdata = X.test.scaled)
RMSE(pred = y.pred, obs = y.test)
## [1] 2.664047
The model seems to be stable and there is no strong evidence of overfitting.
Visualization
tibble(y_test = y.test, y_pred = y.pred) %>%
ggplot() +
theme_light() +
geom_point(mapping = aes(x = y_test, y = y_pred)) +
geom_smooth(mapping = aes(x = y_test, y = y_pred, colour = 'y_pred ~ y_test'), method = 'lm', formula = y ~ x) +
geom_abline(mapping = aes(slope = 1, intercept = 0, colour = 'y_pred = y_test'), show.legend = TRUE) +
ggtitle(label = 'Model Evaluation')
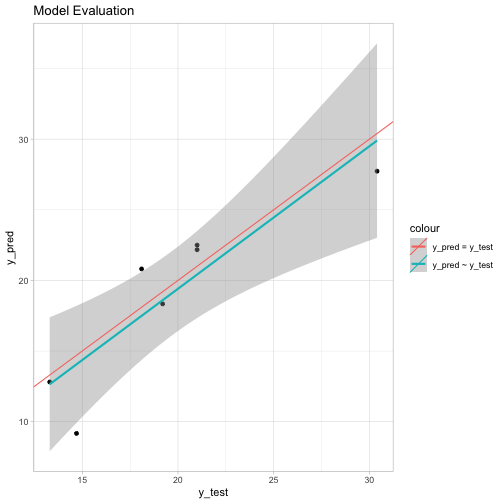
Save Model
Data Pipeline
We define a function which transforms and predicts for new incoming data.
GetNewPredictions <- function(model, transformer, newdata){
newdata.transformed <- predict(object = transformer, newdata = newdata)
new.predictions <- predict(object = model, newdata = newdata.transformed)
return(new.predictions)
}
Save Output Object
# Define Output object.
model.list <- vector(mode = 'list')
# Save scaler object.
model.list$scaler.obj <- scaler.obj
# Save fitted model.
model.list$model.obj <- model.obj
# Save transformation function.
model.list$GetNewPredictions <- GetNewPredictions
saveRDS(object = model.list, file = 'model_list.rds')
Write Plumber Script
This is the basic structure of a Plumber script.
# plumber.R
# Read model data.
model.list <- readRDS(file = 'model_list.rds')
#* @param wt
#* @param qsec
#* @param am
#* @post /predict
CalculatePrediction <- function(wt, qsec, am){
wt %<>% as.numeric
qsec %<>% as.numeric
am %<>% as.numeric
X.new <- tibble(wt = wt, qsec = qsec, am = am)
y.pred <- model.list$GetNewPredictions(model = model.list$model.obj,
transformer = model.list$scaler.obj,
newdata = X.new)
return(y.pred)
}
Expose to API
To expose the model and get predictions we run:
setwd(dir = here::here())
r <- plumb(file = 'plumber.R')
r$run(port = 8000)
We can use a POST
request to obtain predictions.
POST(url = 'http://localhost:8000/predict?am=1&qsec=16.46&wt=2.62') %>% content()
## [1] 22.4974